In today’s world, data is more than just numbers—it’s a story waiting to be told. With tools like Python and Seaborn, you can transform raw data into visually appealing and insightful plots that help you make data-driven decisions. This blog walks you through a hands-on example of creating a professional scatter plot using Pandas, Seaborn, and Matplotlib. By the end, you’ll understand how to bring your data to life with a visually stunning and insightful plot.
We will use a dataset called tips.csv
, which contains information about restaurant bills, tips, smoking habits, gender, and meal times. The goal is to visualize the relationship between the total bill amount and the tip received, while differentiating the data by customer gender.
Let’s understand the code step by step:
Step 1: Importing the Required Libraries (pandas, matplotlib, and seaborn)
import pandas as pd
import seaborn as sns
import matplotlib.pyplot as plt
Pythonpandas
: Helps us read, manipulate, and analyze structured data.seaborn
: A high-level library for creating beautiful and informative visualizations.matplotlib.pyplot
: The foundation for plotting in Python, which Seaborn builds upon.
These libraries work together to give you both the power and flexibility to manipulate and visualize data.
Step 2: Loading the Dataset
tips_data = pd.read_csv('tips.csv')
print(tips_data)
Python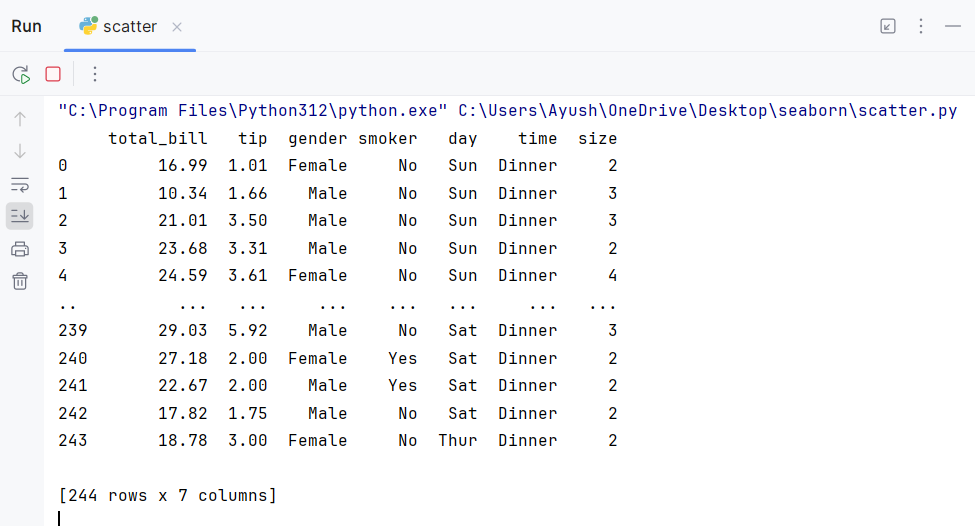
Here, we load the dataset into a Pandas DataFrame called tips_data
using the pd.read_csv()
function. The tips.csv
file contains restaurant data with columns like:
total_bill
: The total amount of the bill.tip
: The tip amount given by the customer.gender
: The gender of the customer (e.g., Male/Female).- Other possible columns like day of the week, time of day, etc.
The print()
function outputs the first few rows of the dataset, helping us understand its structure.
Step 3: Setting Up the Plotting Environment
plt.figure(figsize=(10, 6))
sns.set_theme(style='darkgrid')
sns.set_palette('RdBu')
Pythonplt.figure(figsize=(10, 6))
: Defines the size of the plot. A figure size of 10 inches wide and 6 inches tall ensures the plot is neither too small nor too cluttered.sns.set_theme(style='darkgrid')
: Sets the overall style of the plot. Thedarkgrid
style adds a subtle grid on a dark background, improving readability.sns.set_palette('RdBu')
: Changes the color palette to Red-Blue (RdBu), providing a visually distinct color scheme for gender-based categorization.
For more such content and regular updates, follow us on Facebook, Instagram, LinkedIn
Step 4: Adding a Title
plt.title('Relation b/w total bill and tip', fontsize=20, color='green')
PythonA well-chosen title sets the context for your audience. Here, we define:
- Title Text: “Relation b/w total bill and tip.”
- Font Size: 20, making it prominent.
- Color: Green, which stands out against the dark background.
Step 5: Creating the Scatter Plot
sns.scatterplot(data=tips_data, x='total_bill', y='tip',hue='gender', s=200,alpha=0.7,markers=['*','s'], style='gender')
PythonThis is where the graph is created! Let’s break down the arguments:
data=tips_data
: Specifies the dataset to use.x='total_bill'
andy='tip'
: Define the x-axis (total bill amount) and y-axis (tip amount), respectively.hue='gender'
: Groups the data by gender, assigning different colors to each group.s=200
: Sets the size of the scatter points, making them larger and easier to see.alpha=0.7
: Adds transparency to the points, helping overlapping points stand out.markers=['*', 's']
andstyle='gender'
: Customizes markers for gender categories:- Asterisks (*) for one gender.
- Squares (s) for the other gender.
Step 6: Customizing Axis Labels
plt.xlabel('Total Bill Amount', fontsize=15, color='red')
plt.ylabel('Tips Received', fontsize=15, color='red')
PythonDescriptive axis labels ensure that viewers can quickly interpret the plot. Here, we define:
- X-axis Label: “Total Bill Amount.”
- Y-axis Label: “Tips Received.”
- Font Size: 15 for better readability.
- Color: Red, which contrasts well with the grid.
Step 7: Displaying the Plot
plt.show()
PythonFinally, we use the plt.show()
function to render the plot. This step brings everything together and displays the beautifully crafted scatter plot.
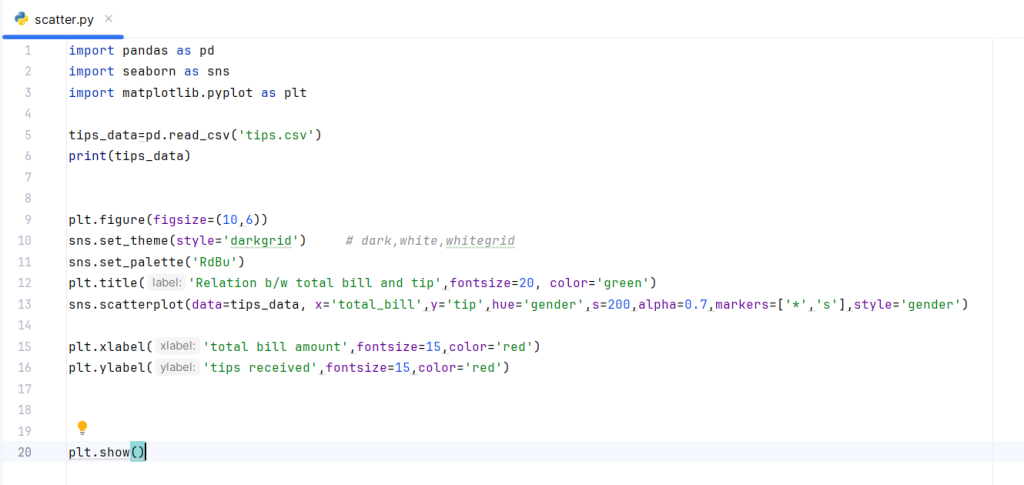
Interpreting the Plot
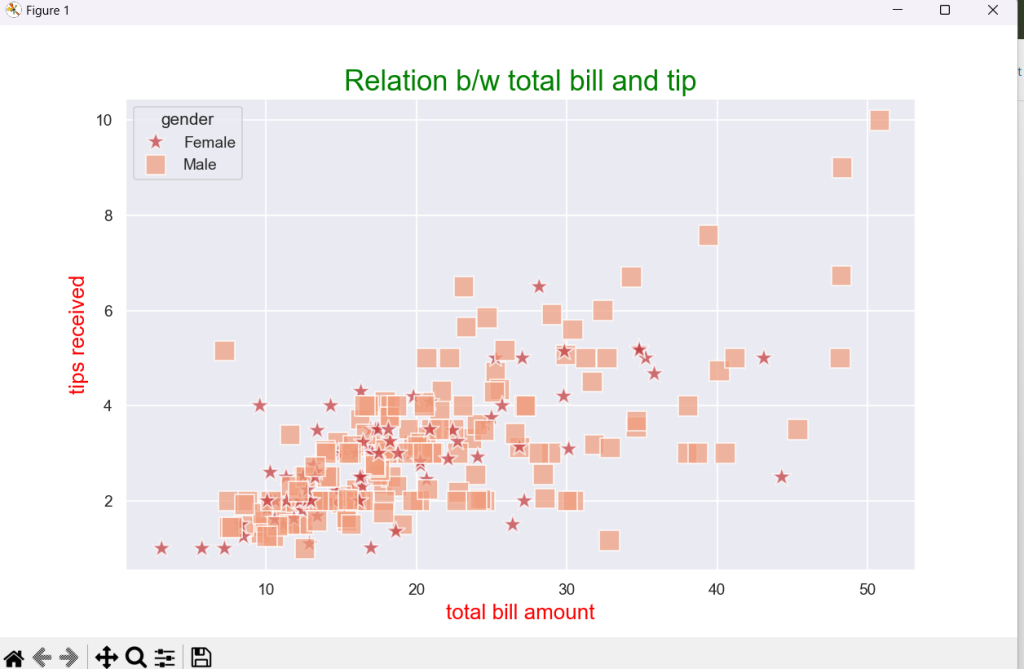
The resulting scatter plot reveals:
- The relationship between the total bill and tips: As the bill increases, tips generally increase too.
- Gender-based trends:
- Each gender is represented with distinct markers and colors, making it easy to compare tipping behavior.
- For example, one gender may consistently tip more for higher bills.
This simple yet effective visualization provides actionable insights for restaurants or data analysts studying customer behavior.
Why This Visualization Matters
- Clarity: The scatter plot clearly shows trends and outliers.
- Customization: Using Seaborn and Matplotlib allows you to customize every detail, from colors to markers.
- Insights: Adding layers like hue and style enables deeper insights into categorical data.
Read more about DATA SCIENCE
If you’re ready to embark on a rewarding career in data science, consider enrolling in a comprehensive course that focuses on Python.
At ConsoleFlare, we offer tailored courses that provide hands-on experience and in-depth knowledge to help you master Python and excel in your data science journey. Join us and take the first step towards becoming a data science expert with Python at your fingertips.
Register yourself with ConsoleFlare for our free workshop on data science. In this workshop, you will get to know each tool and technology of data analysis from scratch that will make you skillfully eligible for any data science profile.
Thinking, Why Console Flare?
- Recently, ConsoleFlare has been recognized as one of the Top 10 Most Promising Data Science Training Institutes of 2023.
- Console Flare offers the opportunity to learn data science in Hindi, just like you speak daily.
- Console Flare believes in the idea of “What to learn and what not to learn” and this can be seen in their curriculum structure. They have designed their program based on what you need to learn for data science and nothing else.
- Want more reasons,
Register yourself & we will help you switch your career to Data Science in just 6 months.
The Complete code
import pandas as pd
import seaborn as sns
import matplotlib.pyplot as plt
tips_data=pd.read_csv('tips.csv')
print(tips_data)
plt.figure(figsize=(10,6))
sns.set_theme(style='darkgrid') # dark,white,whitegrid
sns.set_palette('RdBu')
plt.title('Relation b/w total bill and tip',fontsize=20, color='green')
sns.scatterplot(data=tips_data, x='total_bill',y='tip',hue='gender',s=200,alpha=0.7,markers=['*','s'],style='gender')
plt.xlabel('total bill amount',fontsize=15,color='red')
plt.ylabel('tips received',fontsize=15,color='red')
plt.show()
Python