Data Analyst is one of the most exciting and rapidly growing profiles today. With massive data being generated every second, companies are on the hunt for skilled data analysts who can turn raw data into actionable insights. However, when people think about data science, they usually picture code, algorithms, and complex models. While technical skills are undeniably important, there’s a non-technical side to the field that’s just as critical to your success as a data analyst.
In this blog, you will walk through the essential non-technical aspects of data science, from communication skills to understanding business problems, managing expectations, and thriving in a collaborative work environment. Whether you’re just starting or looking to enhance your data science skills, mastering these non-technical facets will set you apart and accelerate your career.
Read more about DATA SCIENCE
7 Essential Tips For Every Future Data Analyst 2025
1. Understanding the Business Problem: The Key to Relevance
One of the first things every data analyst needs to learn is that data science doesn’t exist in a vacuum. Companies hire data analysts to solve real business problems, and to do that effectively, you must deeply understand the business context. The questions you should ask yourself before diving into any data project are:
- What problem is the business trying to solve?
- How will the data insights help the business achieve its goals?
- What key performance indicators (KPIs) are relevant to this problem?
For example, if you’re working for an e-commerce company that wants to reduce customer churn, you should focus on identifying patterns in customer behavior that could lead to churn. Without understanding the specific pain points of the business, even the most sophisticated models will fall flat. So, make sure to spend time with stakeholders and understand the business landscape thoroughly.
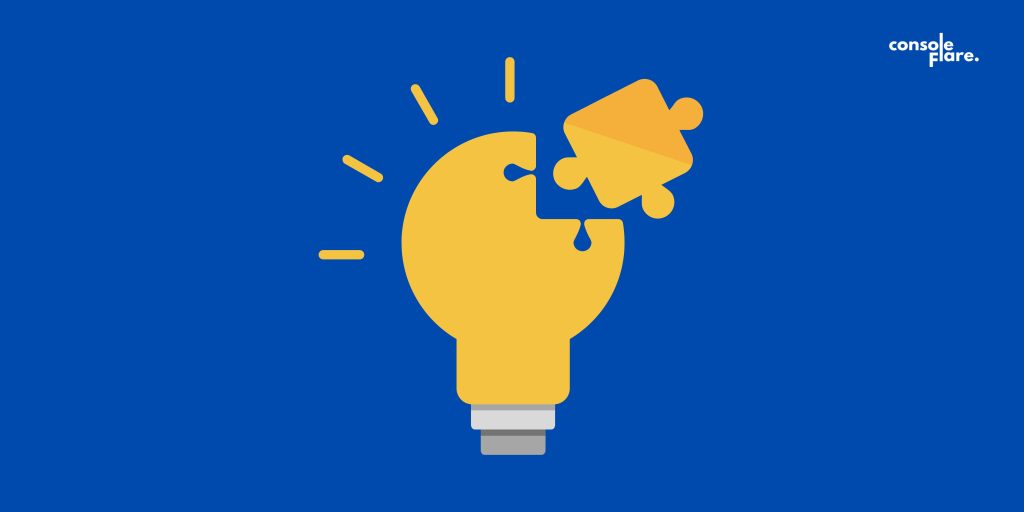
What you need to do: Don’t hesitate to ask questions. Being proactive in understanding the problem will ensure your work is relevant and impactful.
2. Effective Communication: Speak the Language of Your Audience
One of the biggest challenges for any data analyst is translating technical results into actionable business insights. Many budding data analysts get caught up in technical jargon, which often alienates their non-technical colleagues.
While it’s essential to be proficient with data manipulation and statistical techniques, being able to communicate your findings clearly and effectively is what ultimately makes your analysis valuable. You’ll often be presenting your results to managers, clients, or stakeholders who may not have a technical background. Instead of explaining how your gradient boosting model outperformed the random forest, focus on the outcome: “By implementing this model, we can predict customer churn with 85% accuracy, allowing the marketing team to target at-risk customers.”
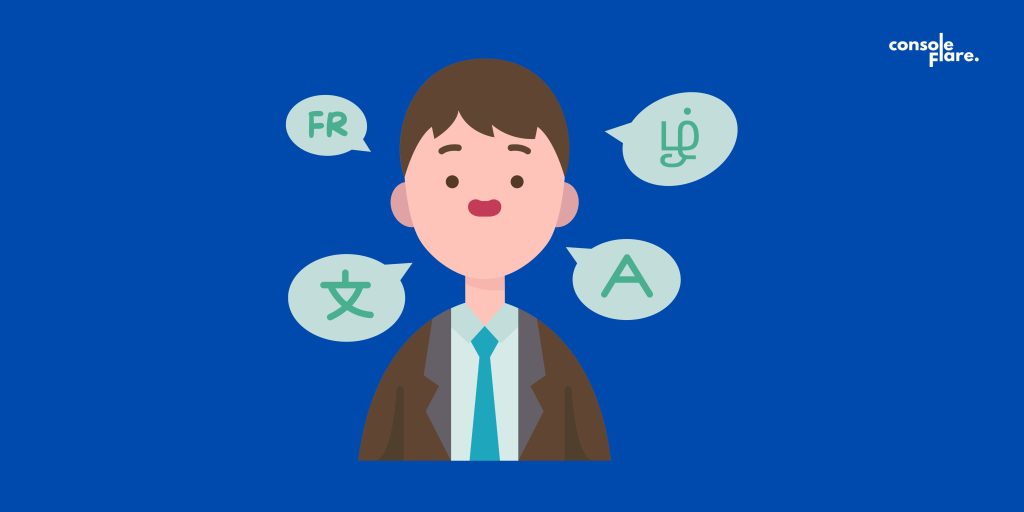
Tips for Effective Communication:
- Know your audience: Tailor your message depending on whether you’re speaking to engineers, marketers, or executives.
- Be concise: Avoid overwhelming your audience with technical details unless they ask for them.
- Use visuals: People love data visualizations. Tools like Power BI, Tableau, or simple graphs in Python’s Matplotlib can make your findings easier to understand.
What you need to do: Practice delivering presentations to non-technical friends or colleagues and get feedback on how well they understood the key points.
3. Time Management: Prioritizing Impact Over Perfection
In the real world, data science projects often come with tight deadlines. As exciting as it is to build complex models, sometimes a simpler solution that can be delivered on time and meets the business’s needs is more valuable.
For example, instead of aiming for a 98% accuracy rate that could take weeks, delivering an 85% accurate model within a week might be more useful to the business.
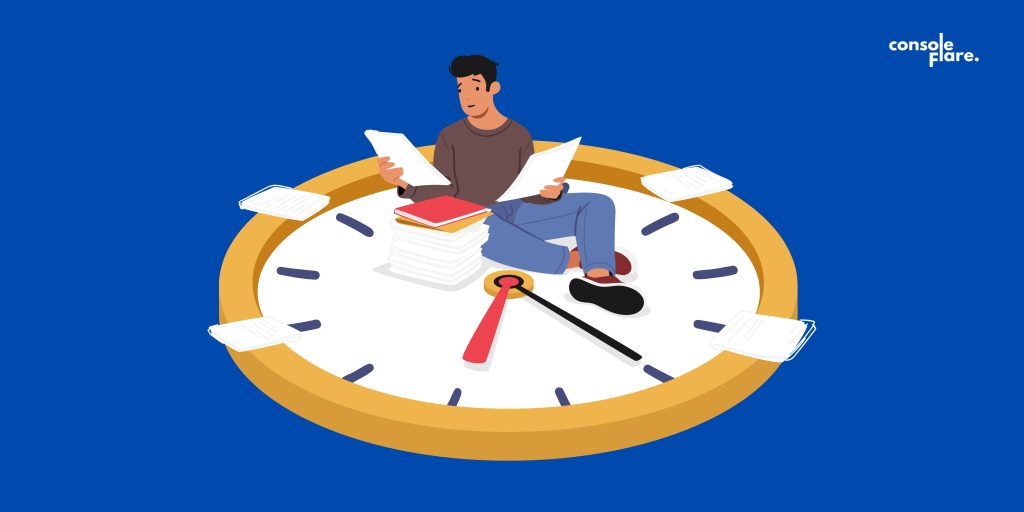
The trick is to balance delivering high-quality work while meeting deadlines. You’ll need to understand when to prioritize speed over perfection and vice versa. This involves working closely with project managers or stakeholders to manage expectations.
Tips for Time Management:
- Break down tasks: Divide your work into smaller, manageable tasks and set deadlines for each.
- Use agile methodologies: Work in iterative cycles where you can deliver smaller insights before working on more complex problems.
- Regular check-ins: Keep your stakeholders updated about your progress to ensure you’re on the right track.
What you need to do: Always leave room for iteration. It’s better to provide a working model with the possibility of refining it later than to spend too long trying to perfect a single version.
You are reading: For Every Future Data Analyst: 7 Essential Tips
4. Adaptability: The Only Constant in Data Science is Change
The data science field is evolving rapidly. New tools, libraries, and techniques are introduced frequently, and what’s faster today might be slower tomorrow. Adaptability is the key. Beyond keeping up with technical changes, you’ll often be working with changing business requirements. Flexibility in your work approach and continuous learning are essential to grow in this environment.
While mastering Python and SQL is essential today, tomorrow you may need to learn PySpark for big data projects or start using cloud-based machine learning platforms like Azure & ML. Successful data scientists aren’t those who know one tool inside out, but those who can quickly learn new tools when needed.
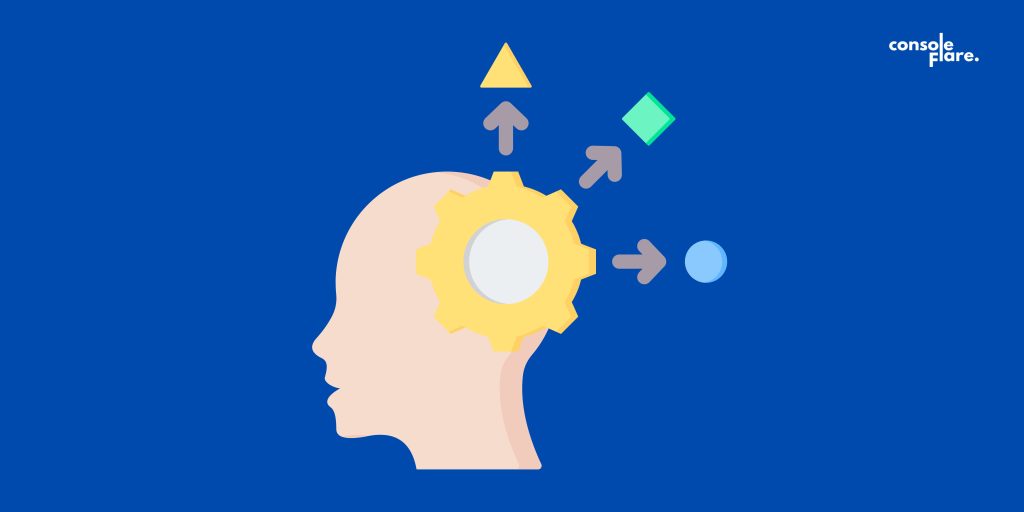
What you need to do: Subscribe to data science blogs, attend webinars, and participate in online communities to stay updated.
For more such content and regular updates, follow us on Facebook, Instagram, LinkedIn
5. Teamwork: Collaborating with Cross-Functional Teams
As a data analyst, you’ll rarely work in isolation. More often than not, you’ll collaborate with product managers, engineers, marketing teams, and even finance departments. Cross-functional collaboration is a daily aspect of the job, and being a good team player is essential.
Working with these teams requires strong interpersonal skills and the ability to listen. Different teams will bring different perspectives, and it’s your job to bridge those gaps by ensuring that your data insights are aligned with the broader business objectives.
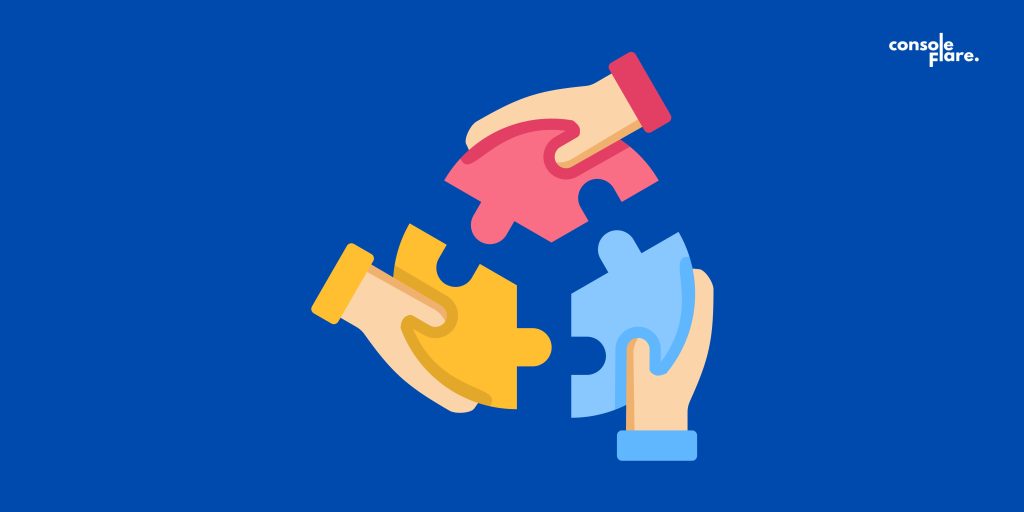
Tips for Effective Collaboration:
- Hold regular meetings: Schedule check-ins with cross-functional teams to ensure everyone is aligned.
- Be open to feedback: Not every suggestion from other teams will make sense from a data science standpoint, but understanding their perspective will help you tailor your analysis better.
- Share your knowledge: Helping your non-data colleagues understand the basics of data science will foster better collaboration.
What you need to do: Always approach teamwork with humility. Collaboration isn’t about proving you’re right but finding the best solution for the business.
6. Managing Expectations: Don’t Overpromise
There’s a common misconception among stakeholders that data science can solve all business problems. This can lead to unrealistic expectations about what your work will deliver. It’s crucial to manage these expectations from the start by being transparent about what can and cannot be achieved with data.
For example, if you’re tasked with predicting customer behavior, it’s important to clarify that no model is 100% accurate and that predictions come with inherent uncertainty. Explain that while your model can provide valuable insights, neither it’s a crystal ball nor you are a fortune teller.
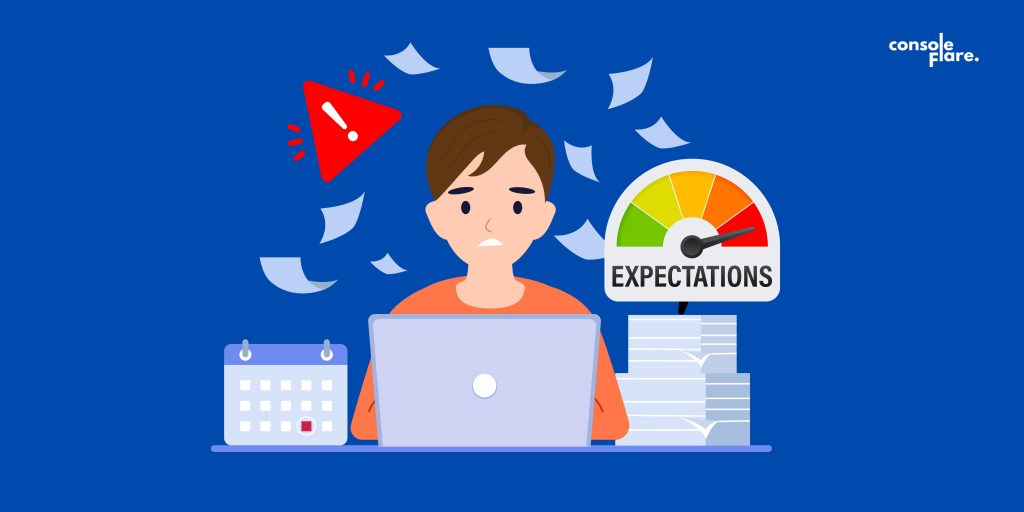
Tips for Managing Expectations:
- Set clear objectives: Make sure stakeholders understand the limitations of data science early on.
- Use clear metrics: Define what success looks like upfront so there’s no confusion later.
- Be honest about uncertainties: Data science is probabilistic by nature, and being transparent about this will save you from future misunderstandings.
What you need to do: Always underpromise and overdeliver. It’s better to exceed expectations than to fall short of ambitious claims.
You are reading: For Every Future Data Analyst: 7 Essential Tips
7. Ethical Considerations of a Data Analyst: The Responsibility of Handling Data
The more advanced data science becomes, the greater the responsibility we have as data analysts to handle data ethically and responsibly. With increasing concerns over privacy, data breaches, and biased algorithms, aspiring data analysts must understand the ethical implications of their work.
As you advance in your career, it’s crucial to ensure that the data you handle is collected, stored, and utilized responsibly. Continuously evaluate the fairness of your algorithms to avoid unintentionally reinforcing bias.
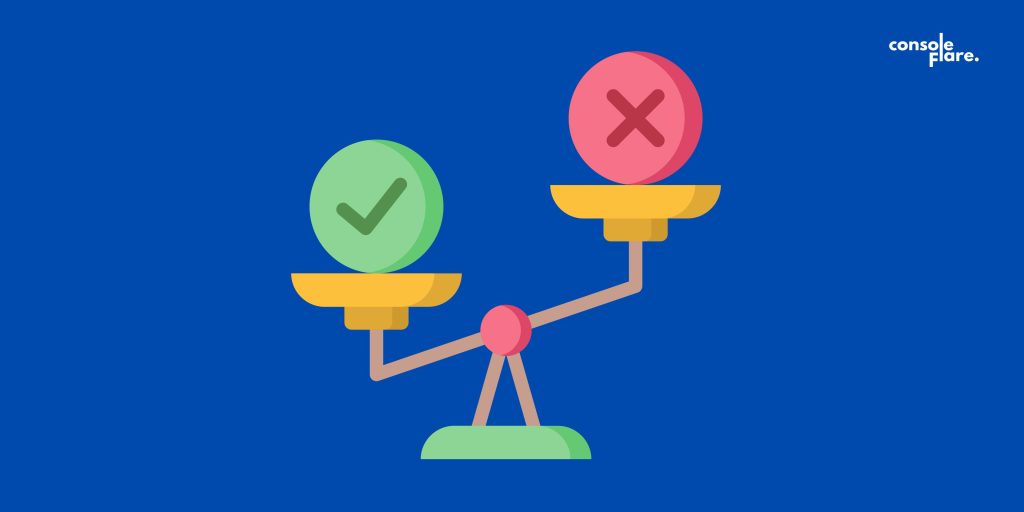
For more such content and regular updates, follow us on Facebook, Instagram, LinkedIn
What you need to do: Keep updated on data privacy laws like GDPR and work closely with your company’s legal and compliance teams to ensure you’re adhering to the best practices.
If you’re ready to embark on a rewarding career as a data analyst in data science field, consider enrolling in a comprehensive course that focuses on Python.
At ConsoleFlare, we offer tailored courses that provide hands-on experience and in-depth knowledge to help you master Python and excel in your data science journey. Join us and take the first step towards becoming a data science expert with Python at your fingertips.
Register yourself with ConsoleFlare for our free workshop on data science. In this workshop, you will get to know each tool and technology that is required for you to become a data analyst from scratch and also which will make you skillfully eligible for any other data science profile.
Thinking, Why Console Flare?
- Recently, ConsoleFlare has been recognized as one of the Top 10 Most Promising Data Science Training Institutes of 2023.
- Console Flare offers the opportunity to learn data science in Hindi, just like you speak daily.
- Console Flare believes in the idea of “What to learn and what not to learn” and this can be seen in their curriculum structure. They have designed their program based on what you need to learn for data science and nothing else.
- Want more reasons,
Register yourself & we will help you switch your career to Data Science in just 6 months.
You were reading: For Every Future Data Analyst: 7 Essential Tips